Insights
What pharma and health techs miss about AI/ML
Written by Daniel Bobear — 2023-02-28.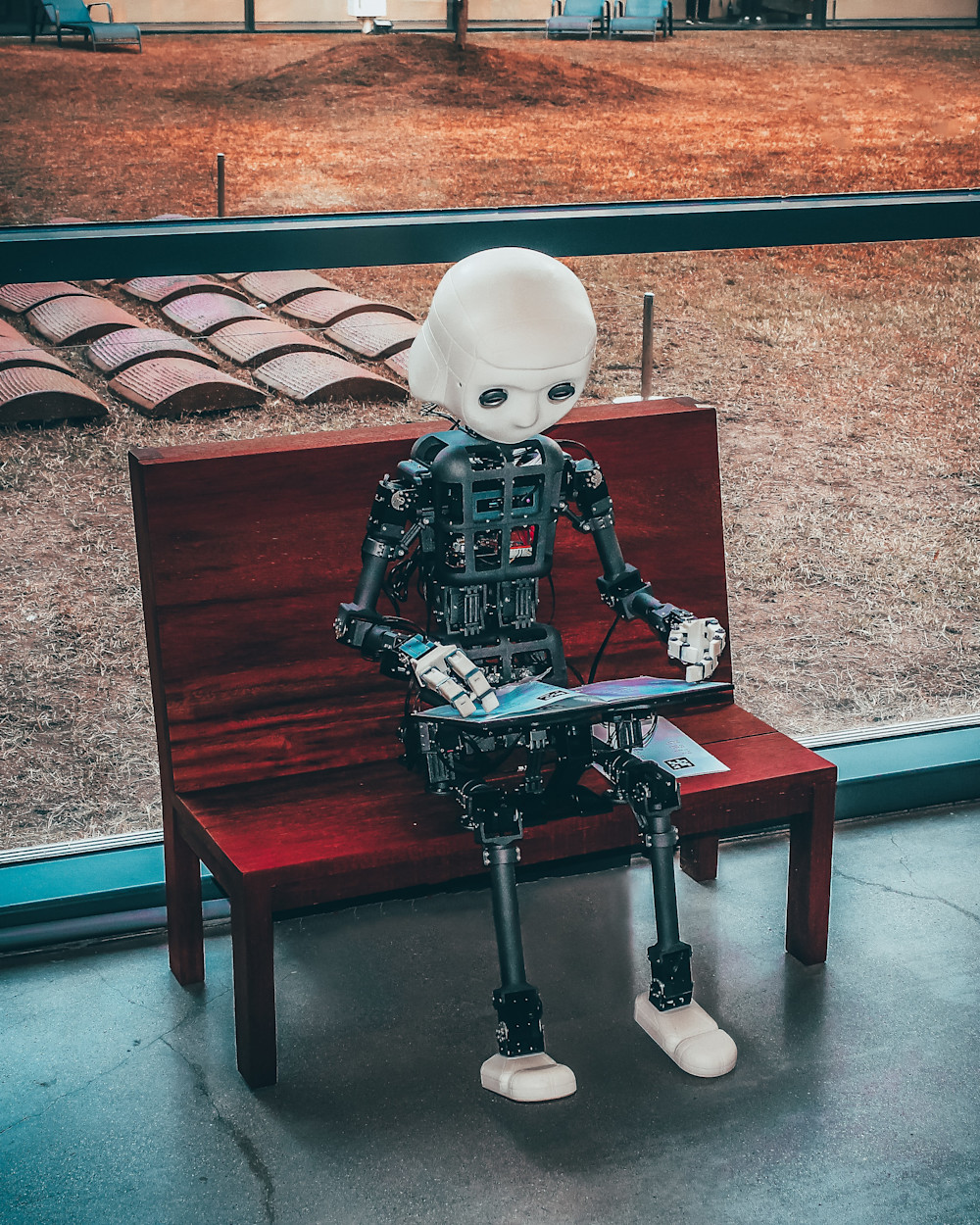
During an internal catchup, the IDEA team stumbled onto a glaring gap: the pharma industry publicly refers to “AI/ML” when describing the power behind new tools applied by pharma and health tech companies—but tends not to go into the specifics of what that means.
Outside of using the broad term describing either artificial intelligence or machine learning as being involved in certain initiatives, the industry does not delve into the actual algorithms nor the reason that certain forms of artificial intelligence are applied, at least publicly. In company press releases and in our experience at IDEA, we see the healthcare industry continuously bucket AI and ML together with the assumption that the computer scientists will do the rest to serve up a useful software. That’s not an effective strategy for truly harnessing the power of these technologies.
First, it is critical for leaders in pharma to understand different types of artificial intelligence and machine learning to understand their best use cases. That requires a conceptual understanding of actual data science. In that sphere, there are six distinct buckets of categorization, Diego Carvallo, a senior data consultant for a boutique consulting firm specializing in AI and machine learning—and the gray area between businesses and machine learning and data—told IDEA in an interview:
Foundational
What needs to be in place before you can even apply data science, building datasets, collecting information
Descriptive
Not so much AI/ML as just understanding what happened as presented in the data
Diagnostic
Connecting different datasets and sources and pieces of information together to come to a conclusion and build out a thesis/model of the relationship between the data that can be leveraged to solve a problem
Predictive
Predicting data science where you can, literally, predict likely outcomes and results using the data
Prescriptive
The evolution from merely predictive to being reliable and robust/sophisticated enough to be able to recommend what to do in a given case based on predictive outcomes
Generative
The ability to create new pieces of data and information (this requires the highest level of sophistication and isn’t even necessary in many business cases)
While it’s not necessary for pharmaceutical leaders and cross-functional business units to know the hard-coding behind each algorithm of the complex healthcare AI systems being developed, it is crucial for them to understand the nuances behind these different applications of data science in order to enable the tailoring of algorithms to the right business problems.
This understanding of the basic uses of data can help leaders make decisions around how to best collect, acquire, and consolidate datasets including, for example, anonymized patient data to position their company in an optimal place among the adoption curve of an area that can lead not only to better patient outcomes, but provide key commercial insights if proper importance is placed on this input data.
“To give you a concrete example, if you think about the delivery chain in pharmaceuticals, there's this issue where the chain isn't always as structured as you'd want it to be,” Carvallo said. “So you might have a great drug, but maybe it’s not delivered to the right person at the right time in the right location. Fixing that doesn't necessarily have anything to do with forecasting or predicting as much as it does understanding your data and bringing datasets together.”
This describes a “diagnostic” model, according to Carvallo, with a powerful business question and a strategy for using data science to achieve a tangible result rather than think an algorithm can just create precise predictive and generative results out of much simpler set of data.
“In this use case, I might look at the people in a specific location, and then overlay that with, for example, a specific pharmaceutical’s sales. And then you might overlay that with socioeconomic factors, or drug availability, to where you could end up seeing, say, a heat map of what is possible to do about this delivery chain problem, which is to say, these are the areas that you need to get your drug into,” he said.
“When you start with the business case in that way, a problem of making sure that the right people have the right drugs at the right location, that is where you start to see really good and practical uses of machine learning and AI and analytics—because you're starting with the business question.”
Furthermore, when it comes to learning algorithms, the output and subsequent insights are only as strong as the input data fed into them. This remains the primary limiting factor to widespread adoption of machine learning processes in pharmaceutical operations due to a few problems. On the industry side, higher-level decision-makers should be fully aware of the fact that passive data collection does not provide enough data to effectively train algorithms that take iteration to optimize. This can be challenging, as proprietary data can be difficult to pry from pharmaceutical companies, patients, and big tech alike to consolidate in a coherent fashion.
From a human standpoint, says Carvallo, the primary barrier to consistent data is a phenomenon known as the “data privacy paradox;” this term describes the general desire of common people to reap the benefits of artificial intelligence, but are hesitant to share their health data with pharmaceutical companies that have commercial interests outside of improving patient outcomes and healthcare system efficiencies. This is an issue that is prevalent across all industries, namely with big tech, but takes on a whole new life when it comes to HIPPA-protected data.
So what can business leaders do? One simple, yet effective way for business decision-makers to realize the potential impact of artificial intelligence on certain healthcare activities is for these leaders to be involved in decisions related to which datasets are emphasized and bolstered. This calls on data scientists to build algorithms to output interpretations in ROI-based terms, but also for pharma leaders to push for more data sharing, standardization, and consolidation.
With broad communication between computer scientists and commercial decision-makers throughout the data collection process, leaders in the industry will have the knowledge they need to make progress on a given issue—not only be more easily trusted, but to facilitate more swift, simplified decisions that can provide healthcare benefits to stakeholders in all facets of healthcare provision and reception.
IDEA Pharma
We work with clients early in lifecycle, crafting a compelling product story and building a best-in-class strategy that helps every molecule reach its potential. It’s what we do best. And there’s nobody that does it quite like us IDEA Pharma